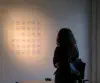
Littérature et numérique
Partners : Algolit, An Mertens, Gijs de Heij
Support : Maison du Livre de Saint-Gilles
Photo credits : Guillaume Slizewicz
An exhibition on poetry, algorithms and artificial intelligence
Glossolalia and Barbarism: the algoliterator by Algolit
The algoliterator is a silicone being that has been tamed by algolit, it feeds exclusively on text. It is a companion for writing, a digital oracle, which makes it possible to escape from the white page, to take on the style of well-known authors and to dress its text with the turns and vocabularies of the greatest novelists. For this exhibition at the Maison du Livre, we have fed the algoliterator with thousands of pages by Guy de Maupassant and Ursula K. Le Guin. It is thus capable of generating Maupassant-Guinnian prose. His way of communicating with us is surprising and brings to light a very machinic poetry. We recognise words, grammar, sentences that sometimes make sense, often don’t and that mimic the way these authors write. The excerpts are not just a random sequence of glyphs, and not yet a coherent and reasoned text. They give us a window into the way algorithms understand our language today.
This version of the algoliterator comes from a generative model developed by OpenAi, called GPT2. This model is based on a programmatic architecture called a neural network, and was built by giving an algorithm thousands of sentences to read, then asking it to understand and generate a language. Although this model has been developed for the English language, and it is possible to adjust it, to refine it by giving it additional data. This is what we have done here so that it can generate sentences in French, in the style of the authors. Despite this adjustment, this fine-tuning, this additional “dressage”, we can see vestiges of the English language in certain turns and grammatical errors (for example on gender chords). Despite the fact that this algorithm was initially considered “too dangerous to be made public” by its creators, it seems, in this version, still in its infancy to be unmasked.
Algolit: https://algolit.net Source code:
algoliterator.clone
La voix au chapitre
La voix au chapitre is a stylistic exercise in representing data sets, particularly the subtitles accompanying the broadcasts of the Brussels City Council.
This representation is in two parts:
The first is a set of cards. Each card represents a word spoken at one of the councils that took place between September 2019 and January 2020 and was then written by the person responsible for subtitling these councils. It was then written by the person responsible for subtitling these councils, and it was chosen to appear on this wall. On the cards, next to this word, you can see the number of times it was spoken. It is also accompanied by an example of a sentence, or part of a sentence in which it was pronounced, like a dictionary, an sms or a haiku. It is a limited, half arithmetic, half human representation of a political dataset.
The second part of this representation is a set of sound files. These files are a digital generation of a voice in French. A technology called text to speech. These files broadcast the words of the cards through a voice-generation model, that we trained using the M-Ai-Lab dataset and Tacotron.
To train a computer to have a voice, it is given a large number of texts in text format, and then the same texts read by one or more humans in audio format. The computer then learns how to read, how to transform a text into sound. It learns step by step and we can, if we want, listen to this learning and see how this generation of voices evolves. In the first stages of training, the voice is still in its infancy and makes mistakes, and through trial and error it becomes better.
In order to give a different representation of the subtitle dataset, we have created a system that affects word diction. Here, the quality of the voice reading the card, the degree of development of the generation model, is related to the number of occurrences of the word in the dataset (the subtitling of the town council). Thus, the more frequent the word, the more advanced the degree of development of the model will be, producing a better and more natural diction of the word. And if the word has not been pronounced much, a low degree of development will be used, leading to an approximate, more artificial pronunciation.